How AI is reshaping the future of energy in buildings
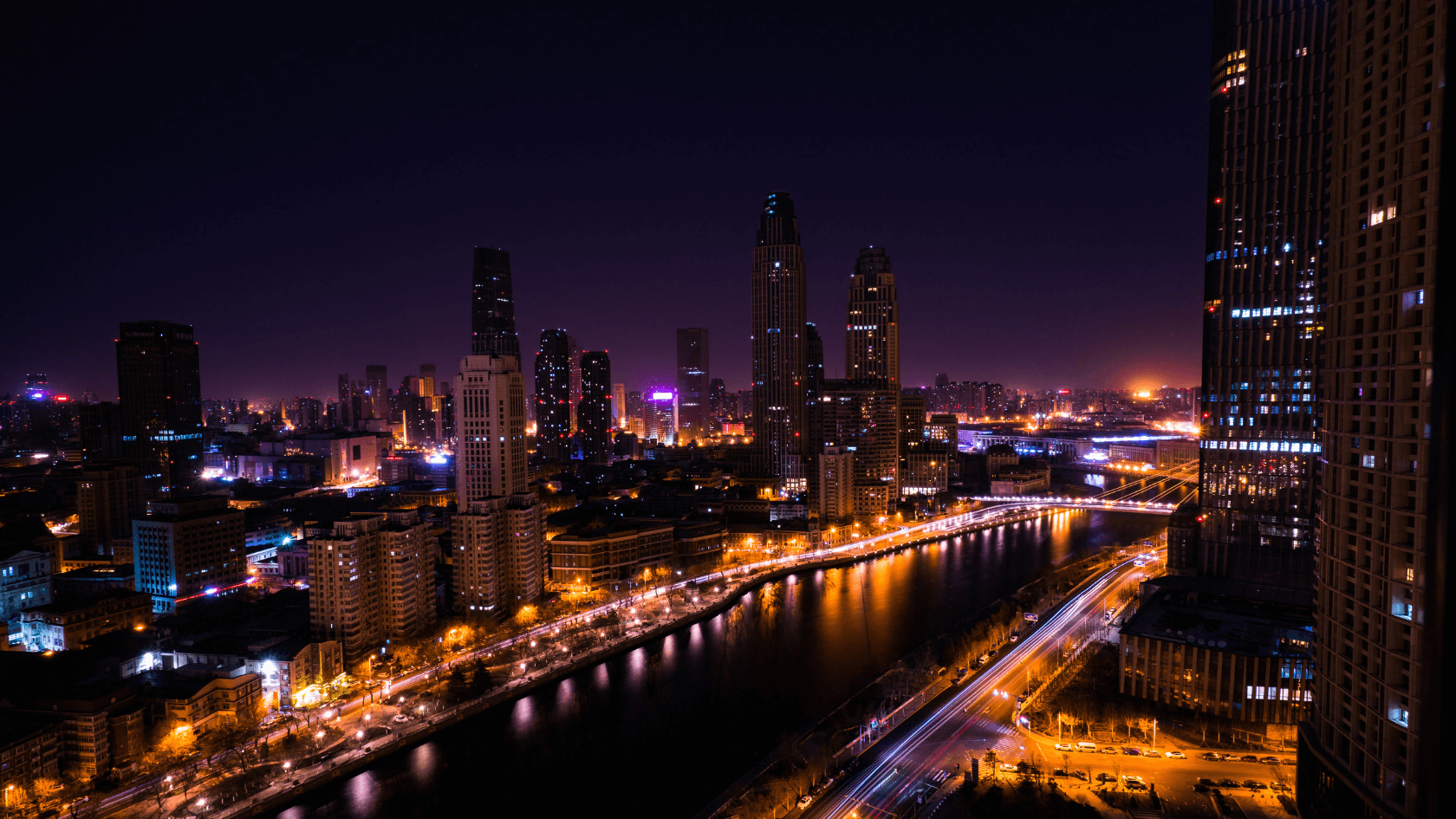
How AI is reshaping the future of energy in buildings
Over the past year, the world has witnessed a transformative shift brought about by generative artificial intelligence. This revolutionary technology has led to unprecedented advancements in multiple different industries and many experts have heralded it as the one of the most significant technological breakthroughs in recent history.
In this article, we’ll look at how other forms of AI are currently revolutionising one of the key infrastructural elements of modern society: the energy sector.
For the past 2 centuries, energy in its different forms has served as a major driver of economic development and improvements in living standards around the globe. However, this intense demand for energy was primarily met through fossil fuel consumption, which led to the extraction of large quantities of carbon stored under the earth’s crust and its release into the atmosphere. This process has been the catalyst of one of the greatest existential threats humanity has ever faced: climate change1.
In recent years, as more and more scientific evidence started to highlight the urgency and severity of a warming climate, saving energy has become a primary focus and is now on the agenda of most public and private organisations around the world. Propelled by vast amounts of computing power and with more and more data available, several AI-based solutions have started appearing in the energy field.
This is the first of a series of posts in which I’ll provide a general overview, as well as some deep dives, on different topics and technologies at the intersection between AI and energy. In this first article, the focus will be the optimisation of energy consumption in buildings through the deployment of Machine Learning models.
The difference between Artificial Intelligence and Machine Learning
To avoid confusion, let’s quickly clarify the terms Artificial Intelligence (AI) and Machine Learning (ML), often used interchangeably.
AI is a broader concept in computer science that refers to the creation of systems capable of performing tasks that typically require human intelligence.
ML is a subset of AI that involves the use of algorithms to enable machines to learn from data and make decisions based on that data. Machine learning is one of the key techniques currently used to realize artificial intelligence, but not all AI systems use ML as their underlying technology2. The techniques we’ll be analysing in this post belong to the Machine Learning category.
Now that we’ve clarified the two concepts, we’re ready to embark on our journey. But before we dive into the technical details, let’s take a step back and try to better understand the context of buildings and their importance in our global effort to reduce carbon emissions.
Why are buildings relevant for climate change?
According to the International Energy Agency (IEA), the operations of buildings (i.e. excluding construction) account for 30% of final energy consumption and 26% of global energy-related carbon emissions globally3. This comprises 8% direct emissions in buildings and 18% indirect emissions from the production of electricity and heat used in buildings.

Making buildings more energy efficient, is therefore seen as essential to achieving global climate targets on time. According to the IEA’s projection, implementing energy efficiency could deliver a reduction in annual energy-related emissions of 3.5 Gt CO2-eq, contributing to over 40% of the abatement required to be in line with the Paris Agreement4.
However, the latest reports have shown that global efforts to reduce energy consumption in buildings are falling short of the necessary progress. A significant change in trend, and a considerable effort is required if we are to reach our 2030 targets in the “Net Zero by 2050” scenario.
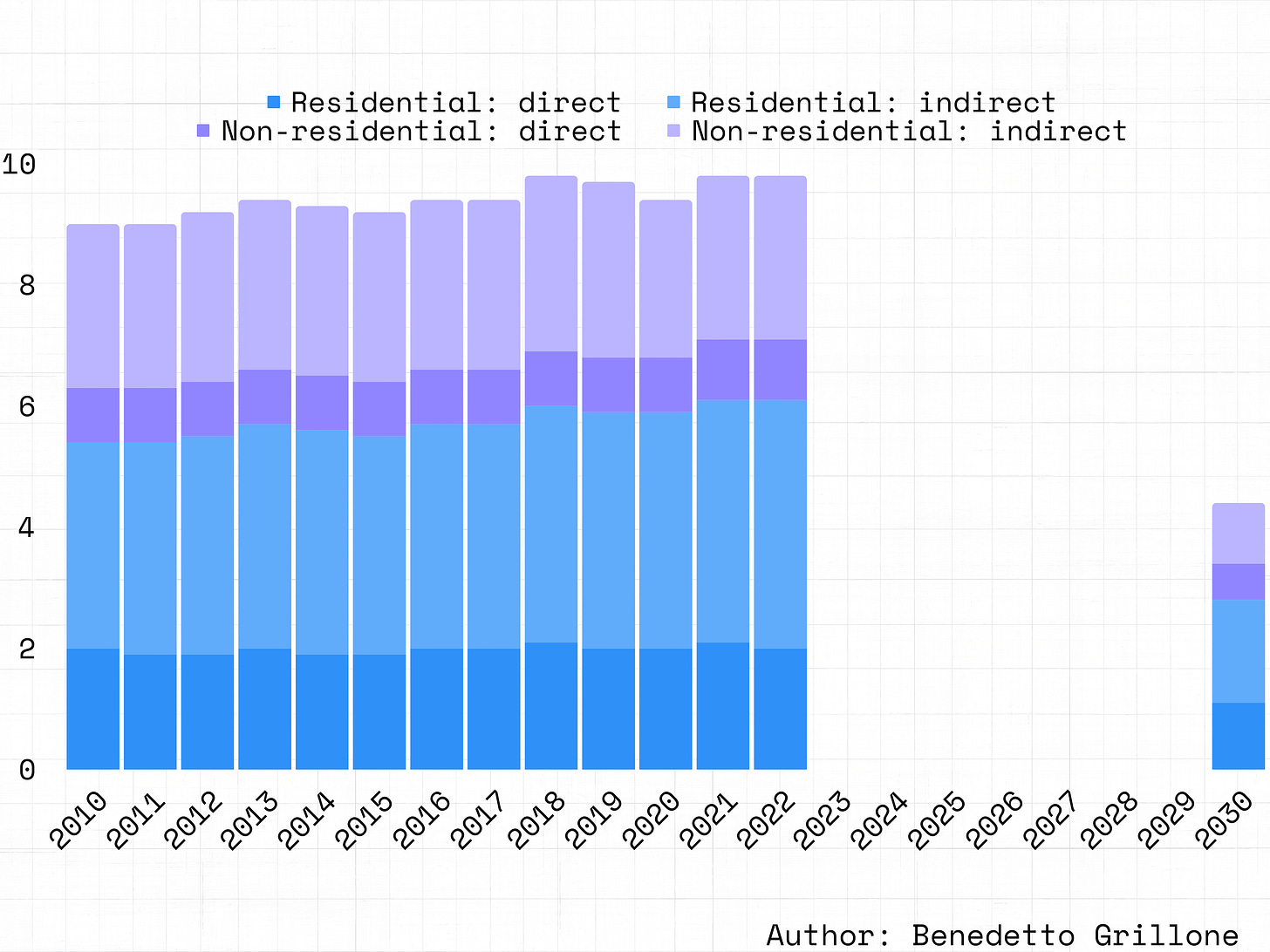
This necessary change of pace could be facilitated by AI-based solutions. This is increasingly feasible due to the rapid digitalisation of buildings and the widespread installation of sensors and meters, which are making the implementation of such solutions possible now and will continue to do so in the coming years. So let’s move on to try and answer the next question: in what ways can AI help us in the ambitious task of decarbonising the buildings sector?
Use-cases for energy consumption prediction
Reducing carbon emissions in buildings is not easy. It is a complex puzzle with many moving parts. One critical piece of the puzzle is creating reliable consumption models of buildings that can then be used to optimise energy use in different ways. Accurate energy consumption modelling allows for timely and informed decisions on energy use, reduce waste, and ultimately contribute to our efforts to achieve net zero energy buildings. This particular area is a key opportunity for AI to make a significant impact.
This all may sound a little bit abstract still, so let’s look at six common use-cases where energy consumption modelling can help with the reduction carbon emissions in buildings.
- Detecting Energy Waste and Consumption Anomalies: Most large buildings are being operated in an inefficient way, leading to substantial quantities of energy being wasted. Energy prediction models can be used to establish the baseline consumption of a building. With that available, anomaly detection algorithms can then be used to continuously evaluate the performance of the building compared to the baseline. Any significant deviations in consumption may indicate problems or inefficiencies, such as equipment malfunctions, which can then be promptly addressed. This is especially important in large facilities, where controlling all systems can be challenging, and small faults can result in significant energy waste if not detected promptly.
- Evaluating Energy Efficiency Measures in Buildings through Advanced Measurement and Verification: Estimating the impact of energy efficiency projects, commonly referred to as Measurement and Verification (M&V), is a complex process. Energy efficiency savings represent an absence of consumption and therefore can’t be measured with an instrument. One way to overcome this issue is by calculating a counterfactual energy model. Counterfactual models are models that are used to consider what the outcome would have been in a situation if a different decision had been made or a different event had occurred. When working with energy efficiency, a counterfactual energy model can be used to estimate the consumption of a building in an alternate scenario where an energy efficiency measure was not implemented. By comparing the real meter consumption and the counterfactual model consumption we can estimate the size of the savings. These models help energy managers to quantitatively assess the impact of implemented interventions and guide future strategies.
- Optimizing Heating, Ventilation, and Air Conditioning (HVAC) Control using Model Predictive Controllers (MPCs): Air conditioning systems can make up a large part of the overall energy consumption of a building. This means that building consumption can be significantly be reduced just by controlling these systems in an optimal way. However, depending on the size of the building, these system can be made of hundreds of components and controlling them can be a challenging task. Model Predictive Controllers (MPCs) are predictive models that aid in making optimal control decisions to balance the delicate interplay between maintaining indoor comfort levels and responding to information coming from building sensors, outdoor weather and the state of the electricity network. This not only leads to energy and carbon savings but also contributes to broader goals such as supporting the integration of renewable energy sources while stabilising the energy grid.
- Estimating the Impact of Demand Side Flexibility Deployment: Demand Side Flexibility (DSF) refers to the practice of modulating demand for energy within a building or facility to match supply availability, primarily during peak demand times. Counterfactual energy models can be used to estimate the flexibility deployment impact, providing a "what-if" analysis that contrasts the actual scenario with an alternative one where the energy demand was not reduced. This has special relevance when calculating flexibility compensations for entities, enabling an accurate representation of the value contributed to the overall energy grid's efficiency and stability.
- Supporting Energy Utilities in Day-Ahead Market Planning: Day-ahead energy markets are based on prediction of the next day's hourly energy demand, a process crucial for energy utilities. Forecasting algorithms play a very important role in predicting these demands, equipping utilities with the insights they need to participate in energy market auctions. By doing so, they can determine the amount of energy they will supply and the corresponding price. Thanks to the accurate estimations of these algorithms, optimal resource allocation is ensured, meaning that high CO2 intensive energy sources, such as natural gas, can be kept on standby when they're not needed, significantly lowering buildings’ indirect carbon emissions.
- Characterising Building Envelope Through Inference Models: A building envelope consists of the physical elements of a building that separate its interior from the exterior environment, such as walls, roofs, and windows. Gaining insights on the quality of a building envelope can highlight areas of inefficiency, such as heat loss through poorly insulated walls, guiding targeted improvements. Energy consumption models can analyse historical energy consumption data to automatically detect poorly insulated buildings, reducing need for ad-hoc energy audits and significantly lowering time for these analysis.

Each of the applications described serves a specific purpose, contributing towards energy optimisation and emission reduction in the built environment. They underline the general value and potential of Machine Learning algorithms in the energy sector, and these examples are just the tip of the iceberg. As the AI field continues to evolve and be refined, we can expect even more innovative and effective uses of these technologies.
If you want to delve deeper into the topic of how to leverage AI to enhance energy efficiency and sustainability, head to Benedetto's Substack.
Notes & sources:
1. If you’re interested in a detailed and well-written account of the existential threats humanity is facing, you might want to check out The Precipice by Toby Ord
2. For a more detailed analysis, see Google's overview of AI vs. ML
3. Source: https://www.iea.org/energy-system/buildings
4. Source: https://www.iea.org/reports/multiple-benefits-of-energy-efficiency/emissions-savings